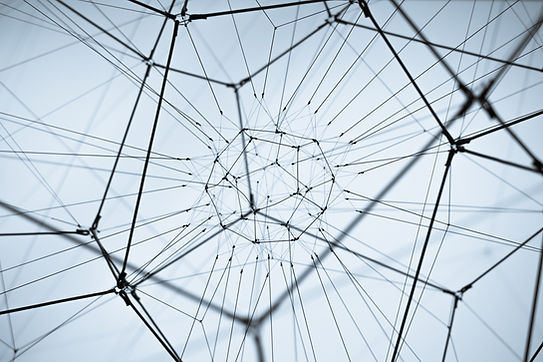

"ALFA"
#Artificial Intelligence for Smart Diagnosis in Building AutomationIm Projekt
ALFA promotes synergies between model-based and ML diagnostic methods in connection with fault diagnosis in building automation. One aim is to alleviate the so-called 'cold start problem' (generally a lack of data for newly commissioned buildings). To this end, simulations are used to generate an ML model. By taking a critical look at the interpretability of ML diagnoses by XAI for transparent decision-making processes and other synergies, new standards are being set in building automation.
Das Projekt ist gleichzeitig eine BRIDGE Kooperation, was bedeutet, dass Forschung und Industrie eng zusammenarbeiten.
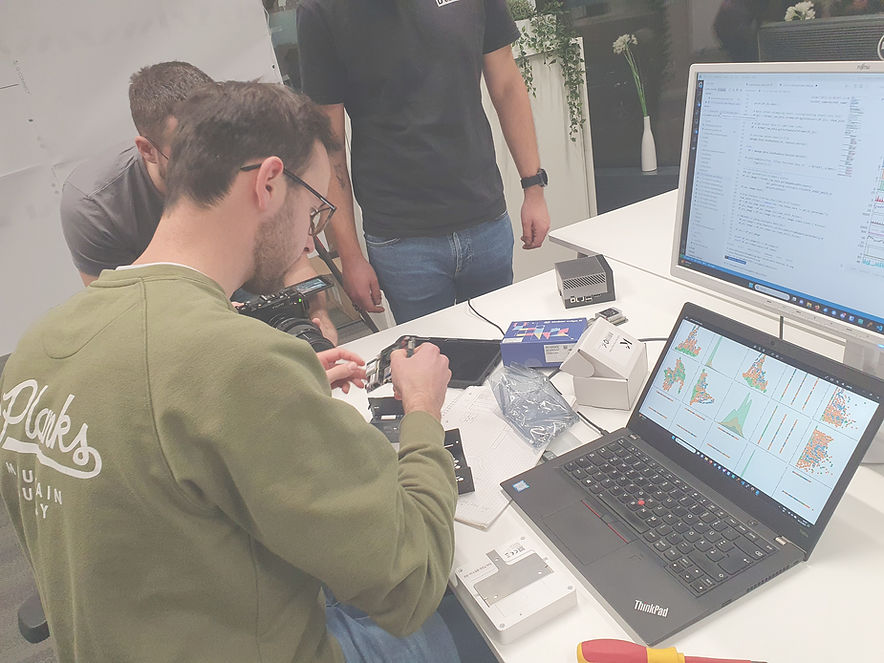
Key Facts
​
Program: FFG (Austrian Research Promotion Agency)
KS 24/26, "Bridge Ausschreibung 2024/01"
​
Duration: 01.11.2024 - 31.10.2027 (36 months)
​Project stakeholders and roles: ​
-
Technical University of Graz - Coordination, Development of AI
-
DiLT Analytics FlexCo - Development of AI
BRIDGE Partners (LOIs secured)
-
Bundesimmobiliengesellschaft BIG - Data & User-Perspective
-
EAM Systems GmbH - Data & User-Perspective
-
Bauer Wärmepumpensysteme GmbH - Data & User-Perspective
-
Radel-Hahn Klimatechnik GmbH - Data & User-Perspective
​​​​​​​
Updates about the project can be found on our LinkedIn channels!!



About the project
The challenge​
Buildings account for a significant portion of energy consumption in the EU and Austria, estimated at 40% and 25%, respectively. Studies have shown that up to 50% of heat pumps operate at only 70-80% efficiency due to a lack of maintenance activities.
By detecting, diagnosing, and repairing faults in heat pumps early on, energy loss can be reduced by approximately 40%. Utilizing smart energy services, such as diagnosis, can enhance energy efficiency and reduce CO2 emissions. Further, diagnosis and repair are crucial components of maintenance that significantly extend the lifetime of products. There are generally two AI diagnosis avenues: knowledge-based, e.g., model-based diagnosis (MBD), and data-driven, e.g., machine learning (ML), approaches. While each research direction has been applied to industrial applications, it becomes crucial to adopt a holistic approach that combines these methodologies to leverage the strengths of both methods, offering a more robust and comprehensive solution.
​​
The project content​
In the project ALFA, we want to foster synergies between model-based and machine learning techniques in the context of fault diagnosis of building automation sector. Based on a set of real-world benchmarks, we will first develop a separate MBD and ML approach in the application domain. Second, we will focus on ways to integrate the methods unique strengths and capabilities. There is generally a lack of data for a newly commissioned building known as the cold start problem. To mitigate this issue, we will exploit simulations based on the MBD model to generate a synthetic diagnostic data set for training the ML model. Additionally, we emphasize the interpretability of ML diagnostics via Explainable AI (XAI), promoting transparency in decision-making processes. Finally, we will explore further synergy opportunities (e.g., incorporating probabilities into MBD based on data from the ML diagnostic process) to set new standards for the accuracy and efficiency of diagnostics in building automation.
​​
The innovation​
​
​Despite the vast amount of research on fault diagnosis using MBD and ML, each of the approaches still has many open research issues that need to be solved for use with HVAC systems of large commercial buildings. While ML methods have been applied to certain aspects of knowledge-based diagnosis, there is currently no framework that effectively combines these two diagnostic routes. Thus in ALFA, we focus on the combination of model-based and ML diagnosis to counteract the approaches’ weaknesses in the context of HVAC systems.
The project goals at a glance
​
Main objective: generate an ML model with real-life data from Heat pumps
​
​Goal areas
​
-
#1 - Goal “Use case identification in Heat Pump Systems and Air Handling Units”
Identifying the key use cases within large-scale Air Handling Units and Heat Pump Systems (thermal power › 70 kW), reflecting the industry's needs in the building domain regarding fault diagnosis.
-
#2 - Goal “Model-based and ML diagnosis:”
Determining and implementing a suitable (1) diagnosis and modeling approach for the model-based variant and (2) ML fault diagnosis in the building domain. This includes in MBD choosing a suitable modeling methodology and diagnostic reasoning strategy for the application
area. In the context of ML, based on the data available an acceptable approach has to be chosen including necessary pre-processing steps.
-
#3 - Goal “Model improvement:”
a) Model-based simulation for the ML cold start problem: Reusing or developing a (set of) diagnostic knowledge-based model(s) in building automation that can be used for simulation. The model(s) must be suitable to generate data that addresses the cold start problem in ML in the building domain and improve the efficiency and effectiveness of the ML model in the initial phase of usage.
b) Interpretability of ML results: Developing and applying a methodology of model-agnostic and/or model-dependent XAI methods to make the decision-making processes of machine models transparent in the building domain.
c) Further model improvement: While we focus on the cold-start problem and the interpretability of ML results in ALFA, we further want to research the combination of the methodologies, i.e., model-based and ML, to improve the diagnostic models. Further synergies between these approaches can be, e.g., the introduction of fault probabilities in the MBD model based on ML learning data or employing hybrid
approaches that integrate ML to assist in identifying and classifying unknown faults.
​​
Sustainability aspects of the project
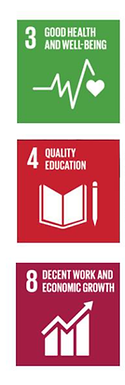
The indoor climate has a direct influence on human and animal well-being. Early detection and correction of faulty building technology and predictive maintainence increases comfort in buildings for people.
The acquired know-how can be utilized in teaching at the TU Graz, and studenrs can be involved as project collaborators, e.g. in master theses or PhDs - or FEMtech internships.
Intelligent software can simplify operations management and reduce the workload for building operators. The academic spin-off DiLT opens up new (international) markets and creates jobs.

As part of the project, real-time FDD (Fault Detection and Diagnostics) services are being developed that could subsequently be applied to systems for generating electricity from renewable energy sources (wind power, photovoltaics, etc.).
The project aims to achieve technological leadership in the field of FDD (Fault Detection and Diagnostics) in the building sector and contributes to the modernisation of the infrastructure through the resulting opportunities.
The use of FDD can reduce the energy consumption of buildings by up to 30 % and increase the system's reliability. With the project results, we are aiming for high scalability and rapid introduction across an entire building portfolio.